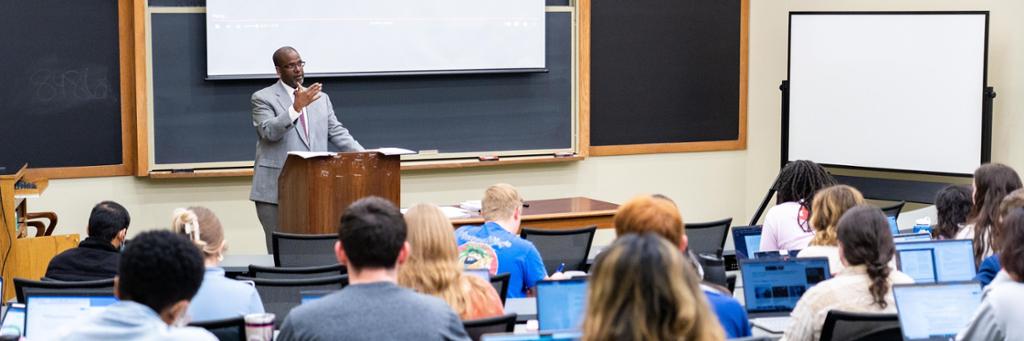
Faculty Guidelines
The guidance below responds to calls from faculty for advice and ideas for how to engage with the recent advances in Artificial Intelligence, specifically a subset that is called “Generative AI” or “GenAI”. We invite you to delve deeper into this concept through the Boatwright Library LibGuide: Generative Artificial Intelligence, which offers an introduction to the concept as well as further reading.
In the sections below, we will use the term “GenAI models” as an umbrella term rather than a particular company (ex. OpenAI’s ChatGPT) or subset (ex. Large Language Models, or LLMs).
Ethical Considerations
There are many ethical considerations regarding the use of GenAI models in academic work.
Perspective and Bias in GenAI: All GenAI models are built with a perspective, which can be shaped by the data that is used to train the algorithm as well as how we use the tool. GenAI models can reflect and amplify existing biases present in their training data. Biases may come from a range of places, including labeling and classification decisions, old data, and skewed representations in datasets. Bias can come in many forms, and here we focus on bias that is prejudicial or unfair. When these biases are not addressed, this can generate problematic results including the propagation of harmful stereotypes. For these reasons, it is important to be attentive to identifying and addressing biased inputs and outputs. It also helps to adopt a mindset of critical evaluation of AI-generated content in which the fairness, accuracy, and consideration of diverse viewpoints and perspectives are examined. AI is built with a perspective, so being attuned to how GenAI models can perpetuate social, cultural, and political biases and harms is important.
Credibility of AI-Generated Content: Developing ways to assess the perspectives, credibility, and reliability of AI-generated information can help with ethical considerations. One way to do this is to check the outputs of GenAI models with multiple reputable and independent sources such as primary literature, academic reviews, original sources, or other resources. For complex or disciplinary-specific information, human expertise and judgment are also important to evaluating content credibility. Notably, the same AI tool may yield different outputs based on the context of the prompt, suggesting the need for continued evaluation of outputs. Finally, it is important to evaluate the context in which the GenAI content was produced. Who made the GenAI model? Do they provide information about how the model was built, particularly the training data? What do their Terms of Service state?
Privacy, Data Protection, and Intellectual Property:
GenAI models need data to learn and generate content. As a result, many of the tools’ licenses (particularly when the tool is free) include the right to keep the data for a period of time or indefinitely. There are three significant issues to highlight.
- An important legal framework that shapes our work is FERPA, the Family Educational Rights and Privacy Act ( see FERPA - Registrar's Office - University of Richmond). We advise that faculty be particularly careful when using GenAI tools that are not provided by the university (including personal subscriptions to tools such as ChatGPT) and have not been approved according to the University Data Security Policy (Policy #IRM-4004). A key component of the policy is that “confidential or restricted information should never be stored with a software or service vendor…unless the University has a contractual agreement with the vendor.” Likewise, to comply with FERPA, please do not include any personally identifiable student information such as name, email address, or student ID to any AI tools that are not provided by the university. Additionally, even if names are removed, it is important to recognize that student work (such as essays, personal reflections, etc.) may still contain identifiable information, such as personal experiences, which students may not want permanently held by an AI company or to contribute to the further development of GenAI models.
- While faculty may choose to submit their own original work to an AI tool, requiring students to submit content to a GenAI model leads to ethical concerns and may violate students’ intellectual property and privacy rights. Our guidance is, therefore, that faculty not require students to enter their own work into an AI tool. We recommend presenting GenAI models as an option, with proper precautions about ethical considerations, but letting the student choose and consent; they should not be penalized for opting out of the use of a GenAI tool..
- The ways in which GenAI tools are built and revised raise questions about who owns the content. There are two simultaneous debates ensuing about GenAI. One is that many of the companies refuse to reveal the data that they used to train their models, with many claims that they were built by violating copyright. Another concern is that feeding copyrighted material into the tools may itself be a violation of copyright law. This can then lead to legal disputes over the ownership of AI-generated content. One recommendation is for faculty to take into consideration the copyright status of any material that they may ask students to use with a GenAI model and to read the Terms and Conditions of the tool, specifically their policy on data. Examples are provided here: Terms of use | OpenAI and Privacy policy | OpenAI.
- Submitting your own written work as a faculty member (for teaching, research, or service) has the same precautions about privacy and data protection as for student work.
Equity and access. Not all AI tools are equally powerful and some of the better tools may require a subscription fee. When incorporating GenAI tools in courses, consider ways to ensure that all students have access to the most useful tools for the task(s).One way is through the new GenAI access program available through IS and the Provost’s Office. (note- this is not yet approved, but if it is approved, we would add clarifying language here).
Support for diverse needs: While GenAI has the potential to support diverse learners (translating text into speech for visually impaired students, for example), we recommend that faculty consider how any assignments using GenAI models could unintentionally disadvantage students with disability accommodations and non-native English speakers. See information below on crafting assignments in GenAI and seek support from the Faculty Hub to ensure the use of inclusive assignments.
Accountability and transparency: The evolving and dynamic nature of GenAI models makes it difficult to know who is responsible if something goes wrong or harm is done.
Academic Integrity: Under the University of Richmond’s Honor Code, students may not use “unauthorized assistance” in their academic work. The challenge is defining, detecting, and assessing such a concept in light of GenAI.
- Defining “unauthorized assistance” varies from course to course. Each faculty member determines what that means for their course and assignments, and we advise that faculty clearly communicate the terms of engagement with GenAI (or other forms of AI) to their students. While some faculty may consider any use of AI-generated work to be an honor code violation, others may allow students to use AI for an assignment or specific parts of an assignment. Before an assignment is due, we highly recommend that faculty remind students of any previously communicated course policies on AI or GenAI.
- Detecting the results of GenAI is challenging and controlling the use of GenAI through detection technology is not currently feasible. While companies and tools for detection are sprouting as fast as new GenAI models, there is currently no reliable detection tool that can be used without a high risk of false positives. The added challenge is that uploading student work into an AI detector may violate privacy policies. For these reasons, we do not recommend the use of AI detection tools at this time. One prominent way to detect GenAI use is to observe the occurrence of “hallucinations” which are made up (untrue) facts and sources/citations. Making up facts and fake sources is a violation of the Honor Code, whether produced by GenAI or not. We recommend following the Honor Code procedures if prohibited use of GenAI on assignments or assessment is suspected.
- Ultimately, encouraging academic integrity is shaped by course design. Transparent and clear assignments, low stakes assessments, scaffolded projects, and ungrading can all be ways to reduce the conditions that lead to unauthorized assistance, whether using GenAI or another resource. Please see “rethinking assessments” below and consult with the Teaching and Scholarship (Faculty) Hub regarding course design and pedagogy.
Rethinking Assessments and Learning Activities
The prevalence of GenAI models has required educators to take a step back and reflect on the potential effects that these tools will have on our students’ learning process. Given the rate at which these tools are evolving and the different ways that our students are adopting them, there isn’t a clear set of principles that can effectively guide us through this uncharted territory. We are all experimenting and learning how these technologies will impact our classes. Some assignments that faculty have created in the past may be less valuable to students’ learning and more likely to be reproduced easily by tools such as ChatGPT. On the other hand, these new tools can also offer unique opportunities to advance student learning and create new teaching opportunities.
One key to effective consideration of GenAI in teaching and learning is early and frequent discussions about GenAI with your students. We recommend that faculty discuss their GenAI policies at the beginning of the term and regularly remind students about them (and any changes) throughout the course. By explaining the rationale behind the policies and encouraging questions, students may better understand the anticipated learning outcomes of the course as well. Discussions about policies, integrity, and the learning process may also build transparency and trust between faculty and students. We recommend that faculty using GenAI models in their teaching emphasize that the goal is to help students use the tools ethically and effectively as a learning tool. It may also be helpful to discuss the value of GenAI in the future workplace.
Below, we present some suggestions that might ground us as we face this pedagogical unknown.
Go back to the basics with Backward Design
Backward Design, a framework highlighted in the book Understanding by Design (Wiggins and McTighe, 2012), proposes that as instructors plan their classes, they start with the end in mind: the desired results. What are the goals and objectives for student learning in our courses? What will a student who has completed a course be able to do at the end of the semester?
Once the learning goals and objectives are clarified, what will be an acceptable evidence of achievement? How will a student demonstrate their learning through assignments and assessments?
Finally, this framework suggests the planning of learning activities and instruction with the goal of helping students gain the knowledge and skills they need to be able to demonstrate learning.
These three steps can all be relevant with Gen AI tools:
-
1. Desired Results / Learning Objectives
There are no easy answers to questions about the ways that GenAI tools might change what students need to know. For many courses, learning objectives will not change - for example understanding what kind of reactions are possible for carbon structures will always be essential for organic chemistry classes. However for other courses, learning objectives might need to be modified. For a writing composition course, as John Warner has been saying for almost two years, “if robots can write a decent English essay, then maybe we shouldn’t be asking our students to write decent English essays”. A recommendation is to ask students to do something else, something more meaningful in their writing than generating five-paragraph essays. Students will continue to need to develop critical thinking and reading skills as well as written communication abilities over the course of their education, but the processes by which they accomplish those objectives likely will evolve in response to GenAI tools.
These conversations about learning objectives and GenAI are ongoing and will require time, reflection, and continued revision.
-
2. Acceptable Evidence of Achievement [Assessments]
AI tools raise the question of what counts as acceptable evidence as learning. If a tool such as ChatGPT can complete a particular assessment at a passable level, it might be that the assessment is no longer acceptable evidence of learning. In reconsidering course assessments such as assignments, exams, and projects, instructors may want to consider integrating elements such as:
- Process-Oriented Tasks: Design assignments that emphasize the process of learning, such as requiring multiple drafts, reflections, and peer reviews. These allow opportunities to focus on formative assessment and the process of writing and thinking. This work can include metacognitive tasks like reflection papers about the process itself.
- Authentic Assessments: Develop “AI-resistant” learning assessments that require critical thinking and real-world application, making it harder for GenAI to complete the tasks independently. Examples include inquiry-based learning, problem-based learning, and scenario-based learning.
- Personalization: Incorporate elements that require personal insights, experiences, or local data, which GenAI cannot easily replicate. These allow students opportunities to analyze, evaluate, or synthesize information obtained from GenAI.
These options do not preclude the use of GenAI tools in assessments and assignments. For example, faculty may choose to ask students to integrate these tools into their writing workflow at specific stages of the process, for example, as a way to plan a project or as an editor of a written draft. In these cases, transparency is a key to success. We advise that instructors ensure that their students understand exactly what are the acceptable (or unacceptable) ways to use a GenAI tool for an assignment or assessment. Instructors might also demonstrate to students the best ways to disclose when they have used GenAI in their work and how to appropriately cite AI-generated content. The library provides guidance on this.
-
3. Learning Activities and Instruction:
We are just beginning to understand how GenAI impacts the process of learning. We know that effort, struggle, and practice are all essential components of acquiring new knowledge and skills. It is important to help students understand that using GenAI tools as a replacement for those three essential components of learning may negatively impact their understanding and skill development.
GenAI tools have shown promise in supporting the learning process when appropriately and thoughtfully used. Below are some ways to consider how faculty might leverage (or avoid) these technologies to help students learn.
- Tutor / Learning Assistant: GenAI models may serve as an on-demand tutor or learning assistant, providing students with immediate feedback and personalized support. By using AI-powered tools, faculty can offer tailored chatbots that can support varying levels of understanding around the clock. This may help address individual learning gaps and reinforce concepts, leading to a more customized learning experience. If used in teaching, it will be important to help students understand the limitations of large language model (LLM) tutor bots. For example, GenAI tools can provide helpful information and support, but they also have constraints, such as potentially generating incorrect or biased information, lacking real-time understanding and context, and being unable to replace the nuanced feedback and personal interaction provided by human teachers. Making students aware of these limitations helps them use LLM tutor bots more effectively and critically.
Expand the Variety of Learning Activities:
- Facilitate Discussions and Simulations: There is promise in using GenAI to help create dynamic discussions, simulations, and problem-solving exercises. For example, GenAI can produce multiple real-world scenarios in science or history classes, allowing students in different groups to engage with different material in a more interactive and immersive way. This can enhance critical thinking and application of knowledge.
- Collaborator for Projects: There is promise in using GenAI tools to promote collaboration in group projects, fostering teamwork and innovative thinking. AI can play specific roles within group work: assist in research, generate ideas, and create mind maps. This collaboration can help students understand the potential and limitations of GenAI, while also enhancing and speeding up their creativity and problem-solving.
Purposeful use or lack of use of digital devices when learning: Faculty may wish to adopt a balanced approach by strategically varying how students engage with technology, including GenAI tools. This may help foster deep thinking and idea creation workflows that benefit from digital efficiencies without being reliant upon them.
Developing Course Policies on AI
Create an AI Policy: We recommend that faculty establish clear guidelines on the acceptable use of AI in assignments and assessments within each course they teach. Course policies related to GenAI should be included in the syllabus and be discussed with students throughout the semester, particularly when assignments are due. Faculty are invited to consult this resource from the Faculty Hub which provides examples of policy statements for syllabi.
To clarify whether and how generative AI tools can be used, we recommend that faculty provide examples of appropriate and inappropriate use cases. Demonstrating to students how GenAI can be used appropriately (or any limitations imposed) in assignments, such as for brainstorming, outlining, and initial drafting creates transparency in the course policies. We also recommend educating students about the ethical implications of GenAI use rather than relying on detection which is unreliable (see section Academic Integrity).
Resources and Support
There are a number of internal and external resources available to faculty interested in using or learning about GenAI for teaching or research.
Internal Resources
The University of Richmond provides the following resources and support to faculty:
- Workshops: The Teaching and Scholarship (Faculty) Hub provides regular workshops on GenAI use both in the classroom and for research and scholarship. See the section on Further Readings and Workshops.
- One-on-One Consultations: Andrew Bell in the Faculty Hub offers one-on-one consultation on how faculty can use GenAI for teaching and research purposes You may contact Andrew Bell via the web or email.
- Digital Pedagogy Cohorts: The Faculty Hub offers a digital pedagogy cohort for faculty to discuss and learn from each other how GenAI can be used in the classroom.
- Collaborative Learning: Faculty may wish to share experiences and strategies with their colleagues to enhance collective understanding and application of AI in education. Consider joining an existing or creating a new Faculty Learning Community (FLC) around GenAI use in teaching and learning (or scholarship).
- GenAI Tools: The University offers access to a number of GenAI tools for free. These include:
- Adobe Firefly for individuals with a university Adobe account.
- Limited free access through the university to a number of OpenAI tools including Chat GPT, Dall E, Whisper, and TTS. For access, contact Andrew Bell in the Faculty Hub. Before fall term or on an ongoing basis, update this with any other approved tools that are offered.
External resources:
Faculty may also find support from a variety of external resources.
- AI Tools: There are a number of AI tools available that faculty can use to experiment with GenAI (some of these require faculty to fund their access through cost centers, grants, or personal funds). These include:
- ChatGPT
- Claude
- Canva Pro
- GitHub Copilot
- Scribe
- Bard
- There are a number of videos and courses on YouTube, Coursera, Udemy, etc. that can be very helpful in learning about generative. There are also a number of books. See the section on Further Readings and Workshops.
Ongoing Updates: We recommend staying informed about institutional policies and updates related to GenAI use.